Ali Haider: AI in Cybersecurity
Introduction
Ali Haider is an industry-recognized cybersecurity judge, mentor, and international award winner. He has traversed various regions, including the Middle East, Europe, and the United States. Ali is a senior cybersecurity consultant for Global Professional Service at Dell Secureworks. His role is instrumental in enhancing visibility and detection and elevating the security posture for the company’s clients. Before joining Dell Secureworks in the USA, Ali had a successful career at Fortune 500 companies such as IBM, STC, and DELL UAE. As a passionate cybersecurity consultant, he has earned several international credentials in networking and cyber security, such as SANS, Cisco Expert (CCIE), CISSP, CISM, and CRISC, to ensure that he stays ahead of intruders, fraudulent users, adversaries, and threats actors.
The Interview
I had the pleasure of interviewing Ali Haider. Our discussion focused mainly on AIWhat is AI? Artificial Intelligence (AI) refers to the simulation of human intelligence processes by computers in an aim to mimic or exceed human cognitive abilities across a range of domains.... More technology’s opportunities and threats for the cybersecurity industry. The copy that follows has been edited for brevity and clarity.
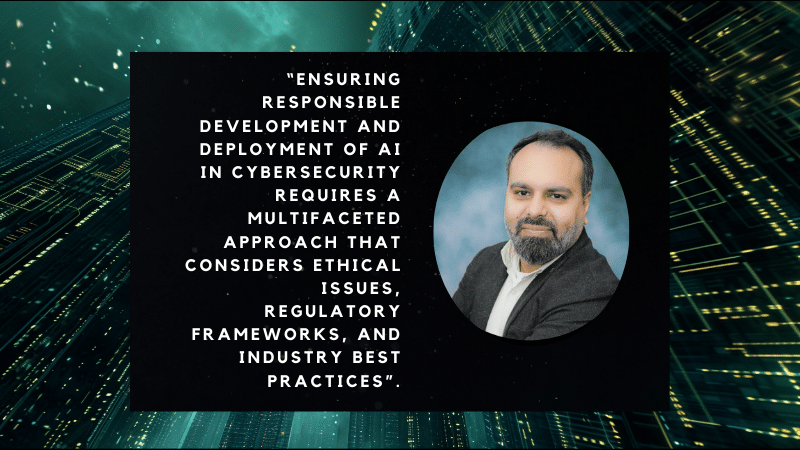
How will generative AI reshape the battlegrounds of social engineering? Can you envision scenarios where Deepfakes and AI-crafted narratives become so sophisticated that traditional detection methods fail?
Ali: Social engineeringWhat is Social Engineering? Social engineering is a manipulative tactic cybercriminals use to deceive individuals into divulging confidential information or performing actions that compromise security. Unlike technical attacks, which exploit... More is a critical challenge in generative AIWhat is Generative AI Generative AI is a subset of artificial intelligence that focuses on creating new content. Unlike traditional AI, which typically analyzes or classifies existing data, generative AI... More through forms like deepfakes and AI-based storytelling. These might change the battlefield by enabling attackers to create authentic fakes, disguising themselves as notable figures, and even manipulating information to trick unsuspected targets.
The consequences of reaching an extent where traditional detection methods are useless in spotting these deepfakes may be severe. For example, perpetrators can exploit deepfake videos or use AI-written messages to represent respected individuals such as company executives and influence victims into providing confidential data or transferring money to fraudster bank accounts.
To adapt social engineering awareness training to keep pace with this evolving threat, several strategies can be employed:
- Employees should receive comprehensive training on the risks associated with deepfakes and AI-crafted narratives, including identifying potential signs of manipulation, such as inconsistencies in content or unusual requests from supposed trusted sources.
- Organizations can conduct simulated social engineering attacks incorporating deepfakes and AI-generated content to test employees’ ability to recognize and respond to such threats.
- Investing in advanced detection technologies specifically designed to identify deepfakes and AI-generated content, such as machine learningWhat is Machine Learning? Machine learning is a subset of Artificial Intelligence (AI) that involves the development of algorithms and models that enable computers to make predictions or decisions based... More algorithms trained to recognize patterns indicative of manipulated media.
- Organizations should establish clear alternate communication protocols for verifying the authenticity of messages and senders, especially those involving sensitive information or unusual requests.
- Given the rapid evolution of generative AI technologies, organizations must continuously monitor developments in this field and adapt their security measures accordingly.
Fostering a culture of cybersecurity awareness and vigilance can further strengthen defenses against this evolving threat landscape.
Is defense outpacing offense? Are security solutions keeping up as attackers leverage AI for malware and phishing? Are there limitations to relying solely on AI-driven defense, and if so, what complementary strategies are needed?
Ali: The cybersecurity landscape is constantly evolving, with defenders and attackers leveraging advancements in AI technology to gain an edge. Attackers are indeed leveraging AI for malwareWhat is Malware? Malware, a portmanteau of "malicious software," constitutes a broad category of software specifically designed to infiltrate, damage, or disrupt computer systems, networks, and devices without the user's... More and phishingWhat is Phishing? Phishing is a type of cyberattack in which attackers send fraudulent communications, or direct people to counterfeit websites in order to trick those individuals into revealing sensitive... More attacks, exploiting its capabilities to develop more sophisticated and targeted campaigns. However, security solutions continuously adapt and integrate AI-powered capabilities to keep up with these evolving threats.
Specific examples of AI-powered security measures successfully thwarting advanced attacks include:
- Advanced Threat Detection: AI-driven threat detection systems can analyze vast amounts of data to identify patterns and behaviors indicative of malware or phishing attempts.
- Behavioural Analysis: AI algorithms can monitor user behavior and network activity to identify deviations from baseline patterns.
- Natural Language ProcessingWhat is Natural Language Processing (NLP)? Natural Language Processing (NLP) is a field that bridges computational linguistics and artificial intelligence (AI), focusing on the interaction between human language and computers.... More (NLP): AI-driven NLP technologies can analyze the content of emails and messages to identify advanced phishing attempts or malicious links.
While AI-driven defense measures offer significant advantages in detecting and mitigating advanced threats, they also have limitations:
- Adversarial Attacks: Attackers can potentially exploit vulnerabilities in AI algorithms to manipulate input data for pattern recognition and statistical analysis and evade detection.
- False Positives and Negatives: AI-powered systems may generate false positives or negatives that can undermine the effectiveness of AI-driven defenses and establish a false sense of security.
- Human Expertise and Oversight: While AI can automate certain aspects of threat detection and response, human expertise and oversight remain crucial. Security professionals play a vital role in interpreting AI-generated alerts and making informed decisions about mitigation strategies.
Complementary strategies to augment AI-driven defense include:
- Human-Centric Approaches: We should not neglect the power of Humans; incorporating human intelligence and intuition alongside AI technologies can enhance threat detection and response capabilities. Security teams can provide context and insights that AI algorithms may overlook, improving overall accuracy and effectiveness.
- Continuous Monitoring and Improvement: Security solutions should undergo regular evaluation and refinement to adapt to evolving threats and address emerging vulnerabilities.
- Multi-Layered Defence: Adopting a multi-layered approach to cybersecurity that combines AI-powered technologies with traditional security measures can provide comprehensive protection against a wide range of threats.
Are automated SOCs the future, or do they risk creating security blind spots? What potential benefits and drawbacks do you see in relying heavily on automated systems for threat detection and response?
Ali: Automated Security Operations Centres (SOCs) hold significant promise in enhancing the efficiency and effectiveness of threat detection and response processes. However, they also pose certain risks, including the potential for creating security blind spots if not implemented and monitored carefully.
Potential benefits of relying heavily on automated systems for threat detection and response include:
- Automated SOCs can analyze vast amounts of data in real-time, enabling rapid detection and response to security incidents at scale.
- AI-driven algorithms can consistently and accurately identify patterns indicative of potential threats, reducing the likelihood of human error and false positives.
- Automated SOCs can free up human analysts to focus on more complex and strategic aspects of cybersecurity operations by automating repetitive tasks and routine security procedures.
- Automated SOCs can provide 24/7 monitoring and alerting capabilities, ensuring potential security incidents are identified and addressed promptly, even outside normal business hours.
However, there are also potential drawbacks to relying heavily on automated systems for threat detection and response:
- Automated systems may struggle to adapt to evolving threats and sophisticated attack techniques that require human intuition and contextual understanding to detect.
- Automated detection algorithms may generate false positives or fail to identify specific types of threats, leading to alert fatigue or security gaps if not properly tuned and calibrated.
- Automated systems may lack the contextual understanding and nuanced judgment that human analysts possess, making it challenging to accurately assess the severity and impact of security incidents.
- Attackers can potentially exploit vulnerabilities in automated detection systems through adversarial attacks, manipulating input data to evade detection or trigger false alarms.
To ensure that automation complements rather than replaces the critical thinking and experience of human analysts, several strategies can be employed:
- Automated systems are designed to augment, rather than replace, human analysts. Therefore, human oversight is essential for interpreting alerts, investigating potential threats, and making informed decisions about response actions.
- Security professionals should receive ongoing training and education to stay abreast of emerging threats and new technologies. This ensures that they can effectively leverage automated tools and apply their expertise to enhance threat detection and response efforts.
- Implementing hybrid approaches that combine automated detection with human analysis can leverage the strengths of both methods. Automated systems can quickly identify potential threats, while human analysts provide context, validation, and decision-making guidance.
- Automated SOCs should undergo regular evaluation and refinement to address performance issues, adapt to evolving threats, and incorporate feedback from human analysts. Continuous improvement processes help ensure that automation remains effective and aligned with organizational goals.
Can XDR, VDR, EDR, and MXDR truly live up to the promise of uncovering hidden threats? What are the real-world challenges of integrating these diverse security solutions?
Ali: Extended Detection and Response (XDR), Vulnerability Detection and Response (VDR), Endpoint Detection and Response (EDR), and Managed XDR (MXDR) solutions hold significant promise in uncovering hidden threats by providing comprehensive visibility and correlation across diverse security data sources. However, achieving the full potential of these unified approaches comes with several real-world challenges, particularly in integrating disparate security solutions and workflows.
One of the primary challenges of integrating these diverse security solutions lies in the complexity of managing and correlating vast amounts of security data generated by different tools and platforms. Each solution typically operates within its own data silo, producing alerts and insights that may not be easily correlated or contextualized without significant manual effort.
Furthermore, integrating these solutions requires overcoming technical hurdles such as interoperability issues, data normalization, and ensuring compatibility across different vendor products. Additionally, organizations must address operational challenges related to governance, risk management, and compliance to ensure that integrated security solutions align with regulatory requirements and organizational policies.
Despite these challenges, there are real-world examples where a unified approach to threat detection and response has yielded valuable insights that might have otherwise been missed.
For example, a multinational financial services organization implemented a unified XDR solution that integrated data from EDR, network security, cloud securityWhat is Cloud Security? Cloud security refers to the measures and strategies used to protect data, applications, and resources stored, accessed, and processed in cloud computing environments. It involves a... More, and threat intelligenceWhat is Threat Intelligence?Also known as cyber threat intelligence or simply threat intel, threat intelligence is the body of evidence-based knowledge that can be used to inform organizations of threat... More sources. By correlating data across these diverse sources, the organization was able to uncover a sophisticated cyber-attack targeting its customer database.
The attack initially went undetected by traditional security solutions but was identified by the XDR platform through anomalous patterns of behavior across multiple endpoints and network segments. The XDR solution provided real-time alerts and actionable insights, enabling the organization’s security team to quickly contain the threat and prevent data exfiltrationWhat is Exfiltration?Exfiltration is the unauthorized transfer of data from a computer or network by an attacker or other entity. In a cybercrime scenario, exfiltration is typically the final stage... More.
Furthermore, the XDR platform’s advanced analytics capabilities allowed the organization to conduct retrospective analysis, uncovering additional indicators of compromise and identifying the attacker’s lateral movement within the network. This holistic view of the attack chain enabled the organization to enhance its incident response procedures and strengthen its overall security posture.
The Ethics Odyssey: How can we ensure responsible development and deployment of AI in cybersecurity while mitigating potential harms? Are there specific ethical frameworks or regulations you believe are needed to guide AI development in this sensitive field? How can we balance the desire for advanced security with the need to protect individual privacy and prevent unintended consequences?
Ali: Ensuring responsible development and deployment of AI in cybersecurity requires a multifaceted approach that considers ethical issues, regulatory frameworks, and industry best practices. To mitigate potential harms and promote ethical AI practices in cybersecurity, several key strategies can be employed:
- Ethical Frameworks and Guidelines: Establishing ethical frameworks and guidelines specific to AI development and deployment in cybersecurity can provide clear principles and standards for responsible behavior. These frameworks should address issues such as transparency, accountability, fairness, and privacy protection. Organizations developing AI-powered cybersecurity solutions should adhere to these principles throughout the development lifecycle.
- Regulatory Oversight: Regulatory bodies should develop and enforce regulations specific to AI in cybersecurity, similar to the EU AI Act, to ensure compliance with ethical standards and protect against potential harm. These regulations may include requirements for transparency in AI algorithms, data protectionWhat is Data Protection? Data protection refers to the practice of safeguarding sensitive information from unauthorized access, disclosure, alteration, or destruction. It involves implementing policies, procedures, and technologies to ensure... More measures, and accountability mechanisms for AI-driven decisions.
- Transparency and Explainability: AI algorithms should be transparent and explainable to ensure accountability and trustworthiness. Organizations should provide clear explanations of how AI-driven decisions are made and enable stakeholders to understand and interpret the rationale behind these decisions.
- Data PrivacyData privacy is the process of safeguarding an individual’s personal information, ensuring it remains confidential, secure, and protected from unauthorized access or misuse. More and Protection: Protecting individual privacy should be a priority in developing and deploying AI-powered cybersecurity solutions. Organizations must implement robust data privacy measures, such as data anonymization, encryptionWhat is Encryption? Encryption converts readable data (plaintext) into a scrambled and unreadable format (ciphertext) using an algorithm and a key. The primary purpose of encryption is to ensure the... More, and access controls, to safeguard sensitive information from unauthorized access or misuse.
- Human Oversight and Intervention: While AI can automate certain aspects of cybersecurity, human oversight and intervention are essential to ensure ethical behavior and mitigate the risk of unintended consequences. Human analysts should actively monitor AI-driven systems, interpret results, and make informed decisions about response actions.
- Ethical Impact Assessments: Conducting ethical impact assessments can help organizations identify and mitigate potential ethical risks associated with AI in cybersecurity. These assessments should consider factors such as bias, discrimination, and unintended consequences and incorporate measures to address these risks proactively.
- Collaboration and Knowledge Sharing: Collaboration between industry stakeholders, academia, and regulatory bodies is essential to promote responsible AI development in cybersecurity. Knowledge sharing and collaboration can facilitate the exchange of best practices, lessons learned, and emerging trends, enabling continuous improvement in ethical AI practices.
In Conclusion
By adhering to ethical frameworks, complying with regulations, and prioritizing privacy protection, organizations can harness the benefits of AI while minimizing potential harm and promoting trust in AI-driven cybersecurity solutions.
To learn more about how Bora can help you with your cybersecurity marketing, contact us today.